When marketing and big data analytics merge, the ability to predict behaviors can be downright eerie.
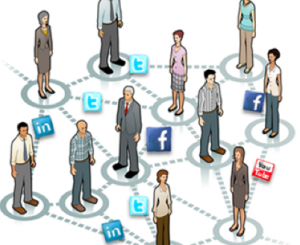
Predicting Customer Behavior
In this article, we will take at how retailing giant Target used predictive analytics to determine if a customer was pregnant and how your company can follow the same principles to identify, predict, and influence customer behavior.
An irate father enters a Target story demanding that they stop sending his daughter promotional information about pregnancy products.
“She’s just a teenager,” he exclaims. “What kind of business are you running? Please stop this nonsense of sending adult material to my young girl!”
The manager of the target is profusely apologetic and says that it will never happen again. He even goes so far as to get in touch with the father a few days thereafter to, once again, make grave apologies on behalf of Target.
Upon receiving the call from the Target manager, the father is now the one who is apologetic. He says: “I’m afraid I am the one who owes you an apology. It turns out my daughter is pregnant.”
How did Target know that the man’s daughter was pregnant even before her own family? Because they know their customer.
How To Use Predictive Analytics to Know Your Customer
While many versions of the story above have been trumpeted about on the Internet, it’s the story behind the story from which we can learn valuable lessons. Just how did Target determine that this young teenager was pregnant?
As Charles Duhigg first mentioned in his piece for the New York Times, two employees in the marketing department of Target asked the following of their statistician, Andrew Pole: “If we wanted to figure out if a customer is pregnant, even if she didn’t want us to know, can you do that?”
Identify a Quality Data Set
Andrew Pole, the statistician, felt uniquely challenged by this question. Up until this point, he had been called upon to perform basic analysis such as: identify families with children and sent them toy catalogs before the holidays.
However, this question presented a challenging new obstacle: Predict behavior that the customer has not disclosed.
The first thing that Mr. Pole had to do was identify a quality data set. Target, long known as an exemplar for knowing their customers, had a lot of historical purchase and behavioral data. Mr. Pole faced one of the biggest problems with big data—it’s too big. There’s simply too much of it.
Fortunately, Mr. Pole was able to identify a data set (a baby shower registry) that included buying habits of women who had disclosed to Target that they were pregnant. He began there.
Identifying a quality data set to be analyzed is one of the first steps in predictive analytics and often one that neutralizes the process from the start. For example, many of our small business customers at The SEO System have said to us: I don’t have “big data” so I don’t have anywhere to begin.
It’s important to note: Lack of big data does not mean no data. Every company has data on their customer. One look at Google Analytics will reveal online patterns to you very quickly.
Do your customers visit your website after you send them an email? Do they respond to your CPC ads? Do they sign up for your newsletter? Do they respond better to questionnaires, how-to articles, or marketing literature? Can they be approached off-line?
While not traditionally big data, this is all actionable data that your small or large business can use to define your customer and reveal patterns that may not have been evident. The important component for your business is to find out what data you have that is important; what is your quality data set?
Identify Customer Patterns
Now that Mr. Pole had identified his initial data set, he began to look for specific buying patterns and behaviors of women from the baby registry data set.
He noticed peculiar patterns that would never be evident with any kind of intuitive analysis. For example, Pole and his team noticed that women began buying unscented lotions around the fourth month of their pregnancy. This piece of data proved invaluable to not only determine if a woman was pregnant, but when the baby was due.
But how do you distinguish a 28 year old women who has purchased unscented lotion simply because she likes it from a 28 year old woman who has purchased unscented lotion because she is pregnant?
This was a challenge to Pole and his team and, to unravel the mystery, they continued to delve deeper into the behavioral patterns of their customers.
They noticed unique patterns in the purchasing habits of pregnant women. The same pregnant women who purchased unscented lotion also purchased supplements such as zinc, magnesium, and calcium. When Pole cross-referenced these purchases along with other seemingly routine products, he realized not only that he could predict the pregnancy status of a woman but he could come very close to predicting her due date.
The question then is this: Does your business have the ability to predict customer behavior based on analytics that already exist?
Again, if you’re not big data, you may default to answering “no” and move on.
But you’d be best-served taking a second look. You do have customer data even if it’s not big data and you need to put on your statistician hat to identify customer patterns.
Presumably by now, you have sent out emails to your customers. Have you analyzed which of those emails gets the best response? What form did it take? Who clicked on those messages (any effective contact program will tell you this)? What steps did those users take after clicking on the message?
Identifying customer patterns is all about asking the right questions about your data sets. It’s about taking a step back to look how seemingly disparate sets of data can work together and allow predictive analysis to take place.
You don’t have to be a statistician to make this happen. You simply have to implement your curious mind and allow it to absorb, cross-reference, and dissect the data available to you.
Creating Actionable Items from Your Predictive Analysis
In spite of Target’s recent failures (like the 2013 Target data breach), Target has long been lauded in the industry for it’s marketing prowess.
In fact, the statistician Andrew Pole saw his position and salary elevated after this predict a pregnancy coup which has been praised and used as a classroom example of how to analyze big data to predict customer behaviors.
This scenario is another example of Target’s marketing mastery. They successfully identified quality data sets to predict buyer behavior to an astonishing level of accuracy.
Then, the time came to create actionable items from their predictive analysis. With this, they made an epic failure of judgment at first: Target created customized mailers filled with pregnancy products to send to the women identified in the predictive analysis.
This led to the situation in the introduction (the angry father) and to many outraged women who felt that Target was spying on them. In his article cited above, Charles Duhigg referred to this as a “public relations disaster”.
Target soon righted the ship as they realized the catastrophe. Rather than sending a coupon mailer filled only with pregnancy products, they threw in other items and made it look as though the pregnancy items were pure happenstance. “Wow,” the expectant mother would think, “a new baby crib next to a lawn mower. How fortuitous for me.”
This approach worked and Target began receiving a financial windfall from their pregnancy predictive analysis.
Predictive analysis provides little benefit without generating actionable items that lead to desired results, such as a sale. In the case of Target, this particular pregnancy case received priority attention because Target had identified the pregnancy time as one in which someone would actually change habits. So, the desired result was not only that the customer buy unscented lotion from Target, it was that the customer become a permanent, life-long Target shopper for all items (such as groceries).
The question companies need to ask is this: Am I using existing data sets to determine customer behaviors and create actionable items that will lead to a desired result?
If a customer makes a purchase after you send a coupon, are you piquing their interest with more coupons? If they are making purchases from one of your CPC ads but not the others, are you creating more of these type of ads? Are they responding to one of your landing pages more than another?
There are often hundreds of questions to be asked to get to the proper actionable items. But once you’ve identified the proper actionable items, the result will be worth the effort.
Richard Cummings
Latest posts by Richard Cummings (see all)
- Indexing Requests Currently Suspended [Google Search Console] - October 15, 2020
- Google Analytics and Google Tag Manager Code:Do I Need Both? - September 19, 2020
- WordPress X Theme:How To Setup Google Tag Manager - September 17, 2020